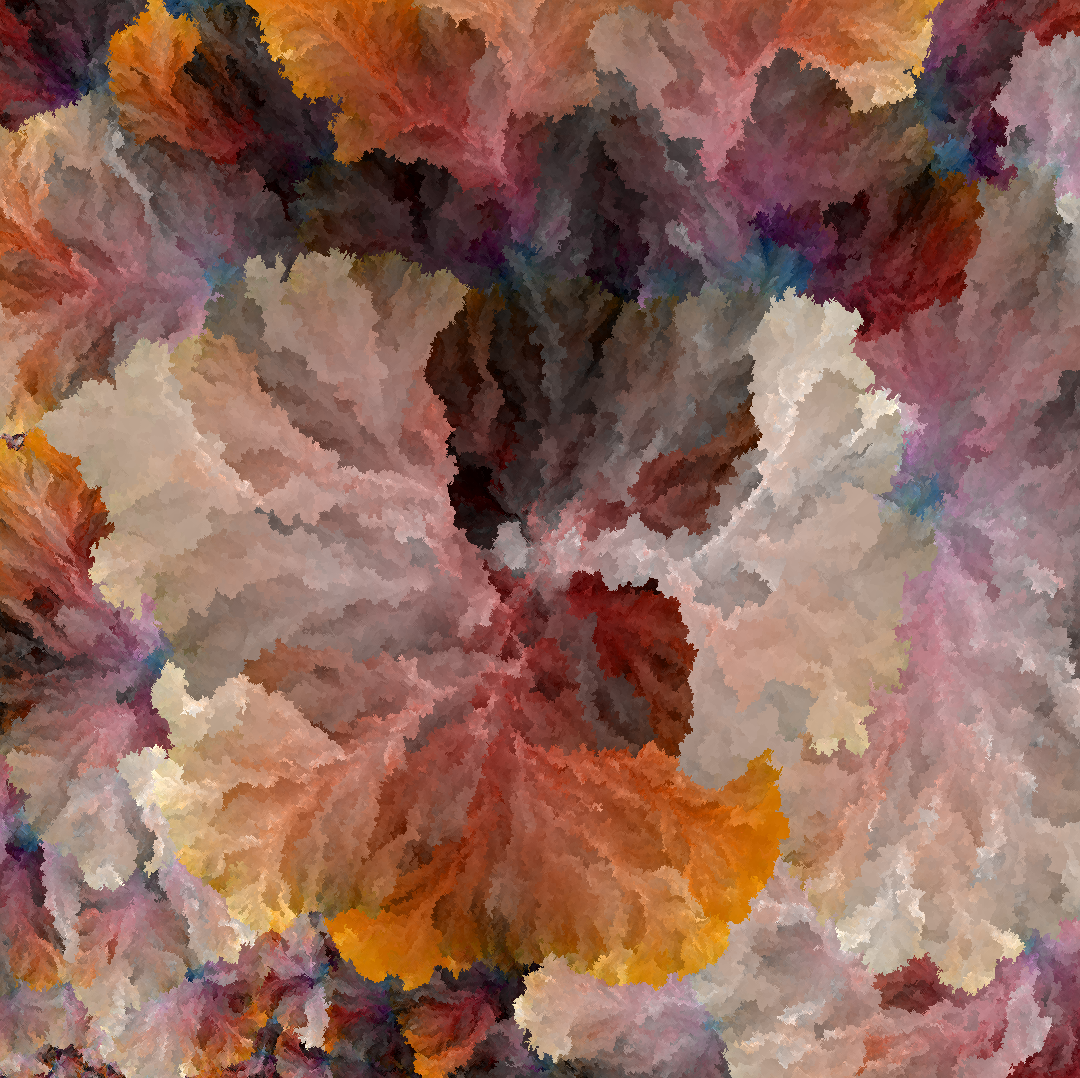
Porting k-d forests to Rust
I recently decided to give a serious go to learning Rust. As my first non-toy project, I decided to port an old piece of code I'd been wanting to dust off anyway: k-d forests. The basic premise of it is to generate images with every possible 8-bit RGB color, by placing each color next to the most similarly-colored pixel placed so far.
The core functionality of that code is an implementation of nearest neighbor search in a metric space, so an obvious place to start is defining a trait to represent a distance metric. The most obvious definition would be something like this:
/// A metric space.
pub trait Metric {
/// Computes the distance between this point and another point.
fn distance(&self, other: &Self) -> f64;
}
I made two enhancements. The first was to allow distances to be represented by an arbitrary orderable type. Often we just want to know whether one point is closer than another, and we can do that faster than actually computing the exact distances. This is true with Euclidean distance, for example, because we can compare distances without taking expensive square roots. This is known as an order embedding of the actual distance.
// An order embedding for distances.
pub trait Distance: Copy + From<f64> + Into<f64> + Ord {}
The second enhancement was to make Metric
generic, to allow computing distances between different types.
This is useful for nearest neighbor search, where the dataset may have more information than the query points (imagine searching for the closest Business
to a GpsLocation
).
The revised Metric
trait looks like this:
/// A metric space.
pub trait Metric<T: ?Sized = Self> {
/// The type used to represent distances.
type Distance: Distance;
/// Computes the distance between this point and another point.
fn distance(&self, other: &T) -> Self::Distance;
}
To actually perform nearest neighbor searches against a set of points, we need data structures that index those points:
/// A nearest neighbor to a target.
pub struct Neighbor<T> {
/// The found item.
pub item: T,
/// The distance from the target.
pub distance: f64,
}
/// A nearest neighbor search index.
pub trait NearestNeighbors<T, U: Metric<T> = T> {
/// Returns the nearest match to `target` (or `None` if this index is empty).
fn nearest(&self, target: &U) -> Option<Neighbor<&T>>;
/// Returns the nearest match to `target` within the `threshold`, if one exists.
fn nearest_within(&self, target: &U, threshold: f64) -> Option<Neighbor<&T>>;
/// Returns the up to `k` nearest matches to `target`.
fn k_nearest(&self, target: &U, k: usize) -> Vec<Neighbor<&T>>;
/// Returns the up to `k` nearest matches to `target` within the `threshold`.
fn k_nearest_within(
&self,
target: &U,
k: usize,
threshold: f64,
) -> Vec<Neighbor<&T>>;
}
These methods could all be implemented in terms of the ones below them, e.g. fn nearest(...) { self.k_nearest(target, 1).pop() }
.
But it will be handy to introduce a special type for accumulating results during a search:
/// Accumulates nearest neighbor search results.
pub trait Neighborhood<T, U: Metric<T>> {
/// Returns the target of the nearest neighbor search.
fn target(&self) -> U;
/// Check whether a distance is within this neighborhood.
fn contains(&self, distance: f64) -> bool {
distance < 0.0 || self.contains_distance(distance.into())
}
/// Check whether a distance is within this neighborhood.
fn contains_distance(&self, distance: U::Distance) -> bool;
/// Consider a new candidate neighbor.
fn consider(&mut self, item: T) -> U::Distance;
}
The nearest*()
methods use a simple implementation that keeps track of the best match seen so far.
The k_nearest*()
methods use a more complicated implementation that keeps track of the k
best matches so far with a priority queue.
Ultimately, all of the NearestNeighbors
functionality can be provided by implementing just one method:
pub trait NearestNeighbors<T, U: Metric<T> = T> {
...
/// Search for nearest neighbors and add them to a neighborhood.
fn search<'a, 'b, N>(&'a self, neighborhood: N) -> N
where
T: 'a,
U: 'b,
N: Neighborhood<&'a T, &'b U>;
}
The simplest possible implementation just does an exhaustive search:
/// A NearestNeighbors implementation that does exhaustive search.
pub struct ExhaustiveSearch<T>(Vec<T>);
impl<T, U: Metric<T>> NearestNeighbors<T, U> for ExhaustiveSearch<T> {
fn search<'a, 'b, N>(&'a self, neighborhood: N) -> N
where
T: 'a,
U: 'b,
N: Neighborhood<&'a T, &'b U>,
{
for e in &self.0 {
neighborhood.consider(e);
}
neighborhood
}
}
Vantage-point trees
Before covering k-d trees, I'm going to go over my implementation of vantage-point trees (VP trees). VP trees are structurally similar to k-d trees, but more general as they work for any metric space, not just . Every node in a VP tree holds a point and a radius, and two subtrees for the points inside/outside the radius.
/// A node in a VP tree.
struct VpNode<T> {
/// The vantage point itself.
item: T,
/// The radius of this node.
radius: f64,
/// The subtree inside the radius, if any.
inside: Option<Box<Self>>,
/// The subtree outside the radius, if any.
outside: Option<Box<Self>>,
}
/// A vantage-point tree.
pub struct VpTree<T> {
/// The root node of the tree.
root: Option<Box<VpNode<T>>>,
}
Searching is fairly simple: we recurse down the tree, using the triangle inequality property of metric spaces to prune subtrees. For a target point , current distance threshold , and a node with point and radius , it's only necessary to search the "inside" subtree when . Similarly, we only need to search the outside subtree when . As a heuristic, we search the subtree that contains the target point first. In code, that looks like:
impl<'a, T, U, N> VpSearch<'a, T, U, N> for VpNode<T>
where
T: 'a,
U: Metric<&'a T>,
N: Neighborhood<&'a T, U>,
{
fn search(&'a self, neighborhood: &mut N) {
let distance = neighborhood.consider(&self.item).into();
if distance <= self.radius {
self.search_inside(distance, neighborhood);
self.search_outside(distance, neighborhood);
} else {
self.search_outside(distance, neighborhood);
self.search_inside(distance, neighborhood);
}
}
fn search_inside(&'a self, distance: f64, neighborhood: &mut N) {
if let Some(inside) = &self.inside {
if neighborhood.contains(distance - self.radius) {
inside.search(neighborhood);
}
}
}
fn search_outside(&'a self, distance: f64, neighborhood: &mut N) {
if let Some(outside) = &self.outside {
if neighborhood.contains(self.radius - distance) {
outside.search(neighborhood);
}
}
}
}
k-d trees
k-d trees have a similar structure. Every node in a k-d tree holds a point , and splits its children into two subtrees based on a coordinate . Points with go in the left subtree, and those with go in the right.
/// A node in a k-d tree.
struct KdNode<T> {
/// The value stored in this node.
item: T,
/// The left subtree, if any.
left: Option<Box<Self>>,
/// The right subtree, if any.
right: Option<Box<Self>>,
}
/// A k-d tree.
pub struct KdTree<T> {
root: Option<Box<KdNode<T>>>,
}
In order to extract coordinate values from points, we define a trait to represent points embedded in Cartesian space:
/// A point in Cartesian space.
pub trait Cartesian: Metric<[f64]> {
/// Returns the number of dimensions necessary to describe this point.
fn dimensions(&self) -> usize;
/// Returns the `i`th coordinate of this point (`i < self.dimensions()`).
fn coordinate(&self, i: usize) -> f64;
}
/// Marker trait for cartesian metric spaces.
pub trait CartesianMetric<T: ?Sized = Self>:
Cartesian + Metric<T, Distance = <Self as Metric<[f64]>>::Distance>
{
}
With that out of the way, the k-d tree implementation is also simple:
impl<T: Cartesian> KdNode<T> {
/// Recursively search for nearest neighbors.
fn search<'a, U, N>(&'a self, i: usize, closest: &mut [f64], neighborhood: &mut N)
where
T: 'a,
U: CartesianMetric<&'a T>,
N: Neighborhood<&'a T, U>,
{
neighborhood.consider(&self.item);
let target = neighborhood.target();
let ti = target.coordinate(i);
let si = self.item.coordinate(i);
let j = (i + 1) % self.item.dimensions();
let (near, far) = if ti <= si {
(&self.left, &self.right)
} else {
(&self.right, &self.left)
};
if let Some(near) = near {
near.search(j, closest, neighborhood);
}
if let Some(far) = far {
let saved = closest[i];
closest[i] = si;
if neighborhood.contains_distance(target.distance(closest)) {
far.search(j, closest, neighborhood);
}
closest[i] = saved;
}
}
}
We can optimize the layout a bit by storing all the nodes together in a flat Vec
rather than separate Boxes
.
Dynamization
k-d trees and VP trees are typically constructed by bulk-loading all points up front. While it's possible to add and remove points from these trees after construction, doing so tends to unbalance the trees. Luckily, there is a general technique to create a dynamic data structure from a static one called "dynamization." k-d forests are a straightforward application of dynamization to k-d trees.
To dynamize insertion, we replace our single tree with multiple trees of exponentially increasing size. As described in the original post, we typically only have to rebuild a few small trees on every insertion. Think of it like incrementing a binary number; only when we have a carry at a particular bit do we have to rebuild the corresponding tree.
/// A dynamic wrapper for a static nearest neighbor search data structure.
pub struct Forest<T>(Vec<Option<T>>);
impl<T, U> Forest<U>
where
U: FromIterator<T> + IntoIterator<Item = T>,
{
/// Add a new item to the forest.
pub fn push(&mut self, item: T) {
let mut items = vec![item];
for slot in &mut self.0 {
match slot.take() {
// Collect the items from any trees we encounter...
Some(tree) => {
items.extend(tree);
}
// ... and put them all in the first empty slot
None => {
*slot = Some(items.into_iter().collect());
return;
}
}
}
self.0.push(Some(items.into_iter().collect()));
}
}
/// Implement NearestNeighbors for Forest<V> whenever it's implemented for V.
impl<T, U, V> NearestNeighbors<T, U> for Forest<V>
where
U: Metric<T>,
V: NearestNeighbors<T, U>,
{
fn search<'a, 'b, N>(&'a self, neighborhood: N) -> N
where
T: 'a,
U: 'b,
N: Neighborhood<&'a T, &'b U>,
{
self.0
.iter()
.flatten()
.fold(neighborhood, |n, t| t.search(n))
}
}
This can be optimized further in the case that multiple elements are inserted at once.
Soft deletion
To dynamize deletion, we do a "soft delete": simply set a deleted flag to true for that item. When the number of deleted items reaches a threshold fraction of the total size, we rebuild the trees without those items. A custom Neighborhood implementation lets us easily skip the deleted items during searches, without having to modify the underlying trees at all! I think that's pretty slick.
/// A trait for objects that can be soft-deleted.
pub trait SoftDelete {
/// Check whether this item is deleted.
fn is_deleted(&self) -> bool;
}
/// Neighborhood wrapper that ignores soft-deleted items.
struct SoftNeighborhood<N>(N);
impl<T, U, N> Neighborhood<T, U> for SoftNeighborhood<N>
where
T: SoftDelete,
U: Metric<T>,
N: Neighborhood<T, U>,
{
fn target(&self) -> U {
self.0.target()
}
fn contains_distance(&self, distance: U::Distance) -> bool {
self.0.contains_distance(distance)
}
fn consider(&mut self, item: T) -> U::Distance {
if item.is_deleted() {
self.target().distance(&item)
} else {
self.0.consider(item)
}
}
}
/// A NearestNeighbors implementation that supports soft deletes.
pub struct SoftSearch<T>(T);
impl<T, U, V> NearestNeighbors<T, U> for SoftSearch<V>
where
T: SoftDelete,
U: Metric<T>,
V: NearestNeighbors<T, U>,
{
fn search<'a, 'b, N>(&'a self, neighborhood: N) -> N
where
T: 'a,
U: 'b,
N: Neighborhood<&'a T, &'b U>,
{
self.0.search(SoftNeighborhood(neighborhood)).0
}
}
Results
This code is available on GitHub here. I had a good experience re-writing it in Rust. I had to ask a couple questions, but got good answers quickly and with a minimum of trademarked Stack Overflow condescension. Prior to this, the only Rust I had written were these 36 lines which were actually just rearranged from existing code, so I was surprised how easy it was to pick up — with the caveat that I've been reading about Rust for quite a while now, even if I hadn't written much.
The new code is quite a bit more general than the old C code, which had the data structure hard-coded to its application of picking nearby colors. Despite the extra levels of abstraction, the Rust implementation is only about 10–20% slower than the C one. And there's even a k-d tree building optimization in the C version that I didn't get around to implementing in Rust, since it requires a bit of tricky interior mutability. That'll probably be the subject of a future post.
The extra generality let me easily implement a couple new features too: you can now use an existing image as the source of colors, instead of the all-RGB-colors cube (that's how I generated the banner image, from this painting). And you can use an image as a target too, placing pixels over their closest counterparts in a given image. Finally, I rendered some videos of the images being generated, which you can find on this YouTube playlist (updated to fix YouTube issues).